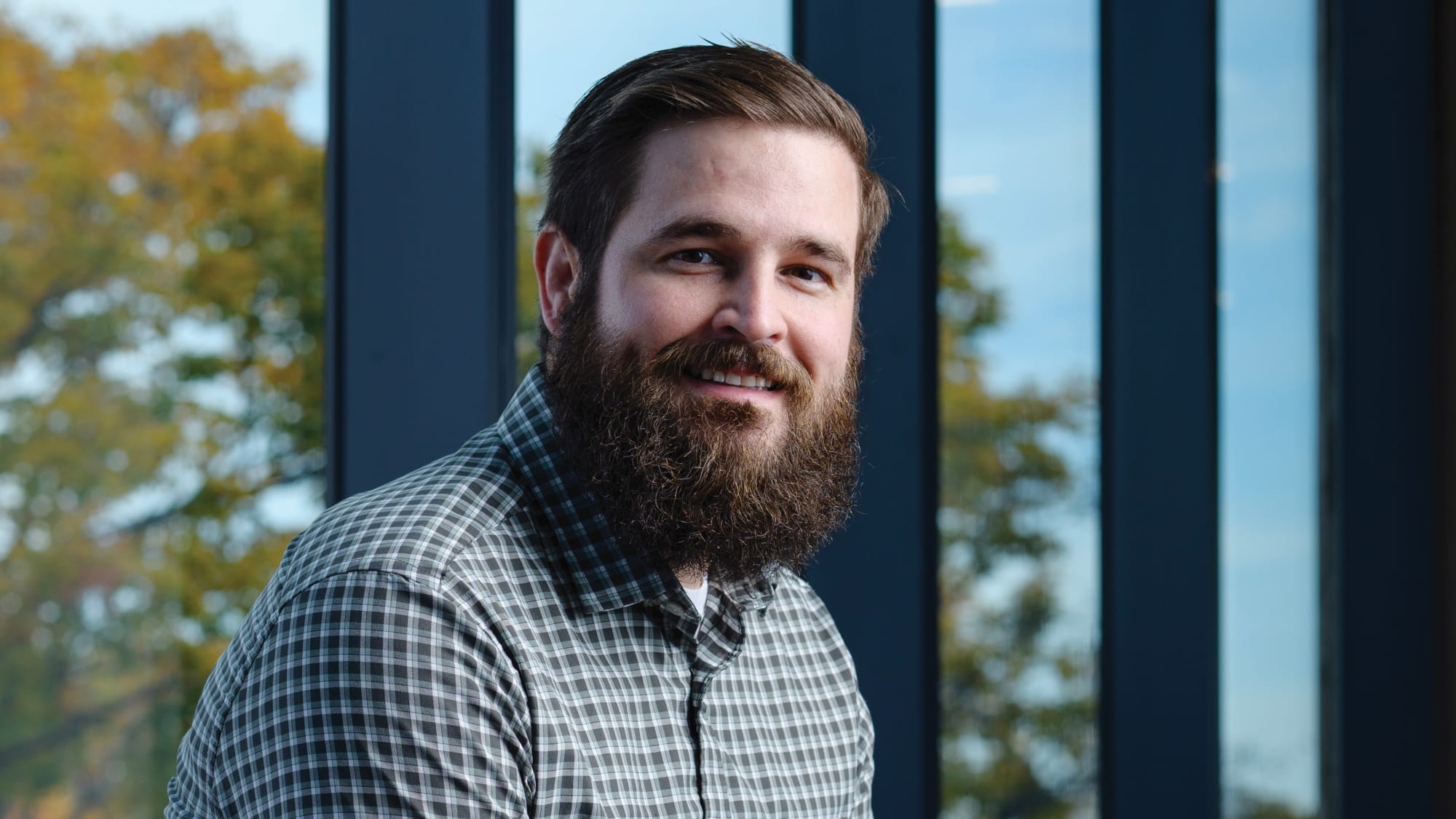
Travis Johnson, PhD, (above) used his bioinformatics and machine learning expertise to collaborate with Brian Walker, PhD, and identify a potential driver of multiple myeloma.
Finding a Signal in Noise
Machine learning enables cancer researchers to sift reams of genetic data and identify a protein potentially powering multiple myeloma.
Matthew Harris Dec 17, 2024
UNLIKE SOME CANCERS, myeloma doesn’t take just one route to roar back after being tamped down by an initial therapy.
The culprit could be chemicals that control how genes are expressed in cells. Sometimes, there are changes within the DNA where genetic information is stored. Or the disorder that comes from the rapid multiplication of myeloma cells leads to genes getting rearranged and even deleted.
Understanding how and why this happens is critical. But the number of variables that drive the recurrence of myeloma explode at a scale our minds can’t fathom.
Two IU School of Medicine scientists — Travis Johnson, PhD, (pictured above) and Brian Walker, PhD — have found a vital connection: Patients with an extra copy of one chromosome also have elevated levels of a protein that myeloma relies upon to usher in its return.
That insight came from cells donated by patients at IU Melvin and Bren Simon Comprehensive Cancer Center. It came with help from a machine-learning algorithm — a tool devised by Johnson, a data scientist, and put to scrutiny by Walker, a medical and molecular genetics professor.
“We used my deep learning algorithm to identify high-risk cells,” said Johnson, an assistant professor of bioinformatics and health data science and the Agnes Beaudry Investigator in Myeloma Research. “We found a marker, which is known to be important. Then, we went hunting for it in 50 other samples, and we found it was associated with relapsed myeloma cells.”
The tandem’s work started in 2019 and isn’t stopping at the discovery of a connection. Using a grant from the cancer center, Johnson and Walker, the Daniel and Lori Efroymson Professor of Oncology, will collaborate with a colleague at Indiana University, Attaya Suvannasankha, MD, and a colleague from the University of North Carolina to test a compound that could potentially block the protein known as PHF19.
To put the protein in their sights, however, they had to sift through terabytes of cellular data — the rough equivalent of about 6.5 million document pages.
By nature, cancer cells make a habit of misapplying orderly rules to copy and divide their DNA. Steadily, cell mutations pile up. This chaos means that changes — even within a single patient — can vary widely.
It was only in the past decade, though, that scientists like Walker had the tools to study what happened within a single cell.
Getting that granular look, however, created a problem of scale.
A sample from one patient may contain more than 5,000 cells. Each of those cells has more than 20,000 genes. The resulting information tallies up to five terabytes, a hefty metric for data. In real-world terms, it’s the equivalent of 800,000 books.
In a modest cancer study of 50 patients, there is enough data to fill 40 million books — far more than the number of cataloged books in the Library of Congress.
“The thing that makes this data the best material to work with is also the thing that makes it terrible to work with,” Johnson said.
To get a handle on it all, Johnson took a freely available public software and rewrote over 400 lines of its computer code. When he was done, his custom-made algorithm sifted through the cell data and another database with clinical information from patients with relapsed myeloma. The result: a tool that could identify batches of cells that correlate with high-risk cancer markers. One of those was PHF19.
Using it, Johnson and Walker found the markers were elevated when the patient had an extra copy of a particular chromosome. One of the markers was a protein that enables the cancer to flip a switch and ramp up its cell division. The protein may even help the cancer repair damage inflicted by drug therapies.
The scientists were able to identify the telltale signs of cancer only with the ability to analyze the enormous set of data — the one so terrible in scale.
Donors help IU School of Medicine recruit talented scientists and invest in bold research to improve how we detect and treat multiple myeloma. Make a gift today, or contact Ashleigh Wahl to learn how to help.
Glossary Terms
Natural Language Processing: A field of AI that deals with how computers understand, interpret and generate human language, allowing them to understand the subtle shades of meaning in how we write or speak.
Generative AI: Models that create new content – such as text, images, or music – by learning patterns and structures from existing data rather than making predictions, as did early AI models.
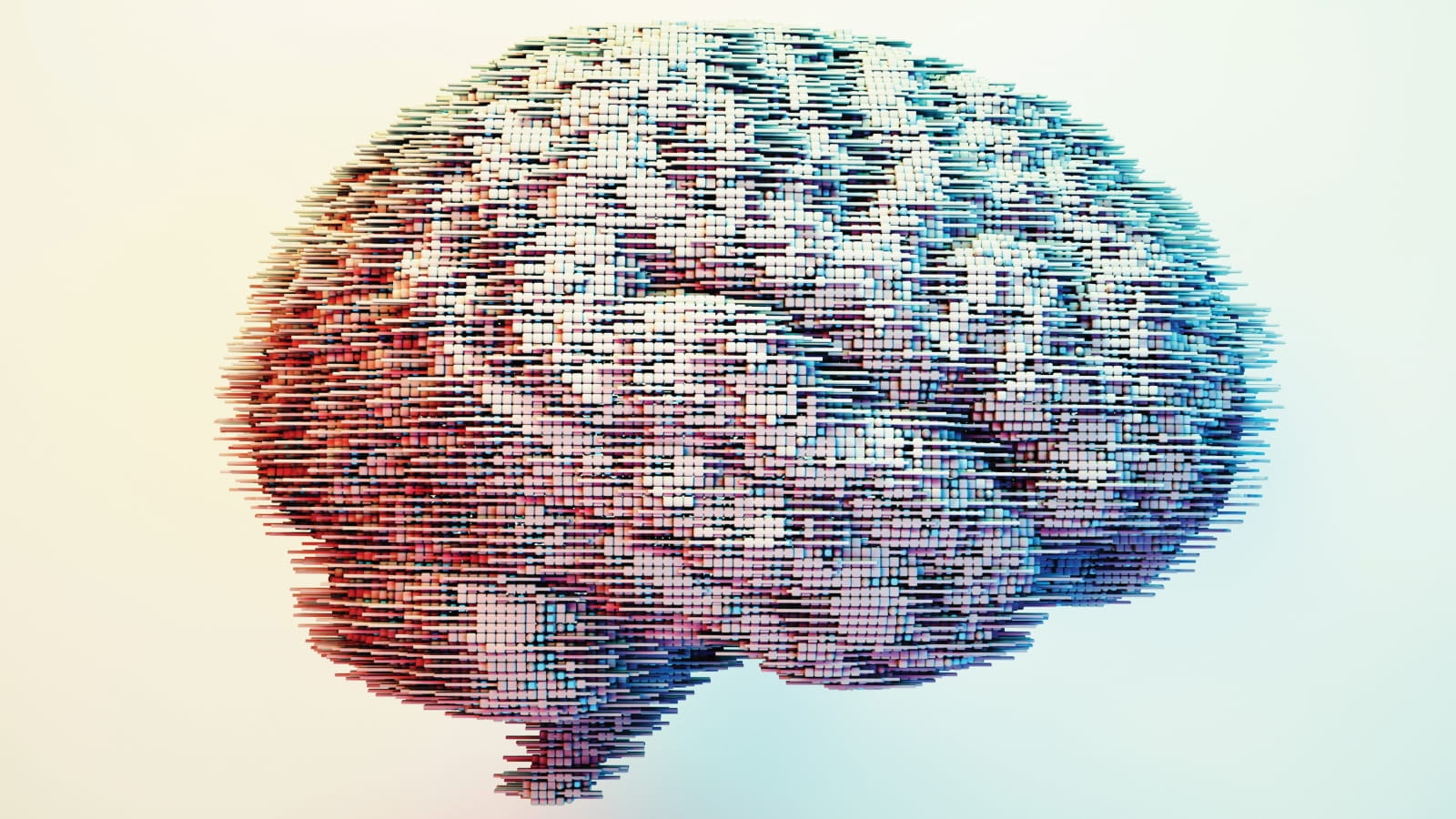
Unlocking the Power of AI
IU School of Medicine embraces a powerful new tool to speed research and treat patients.
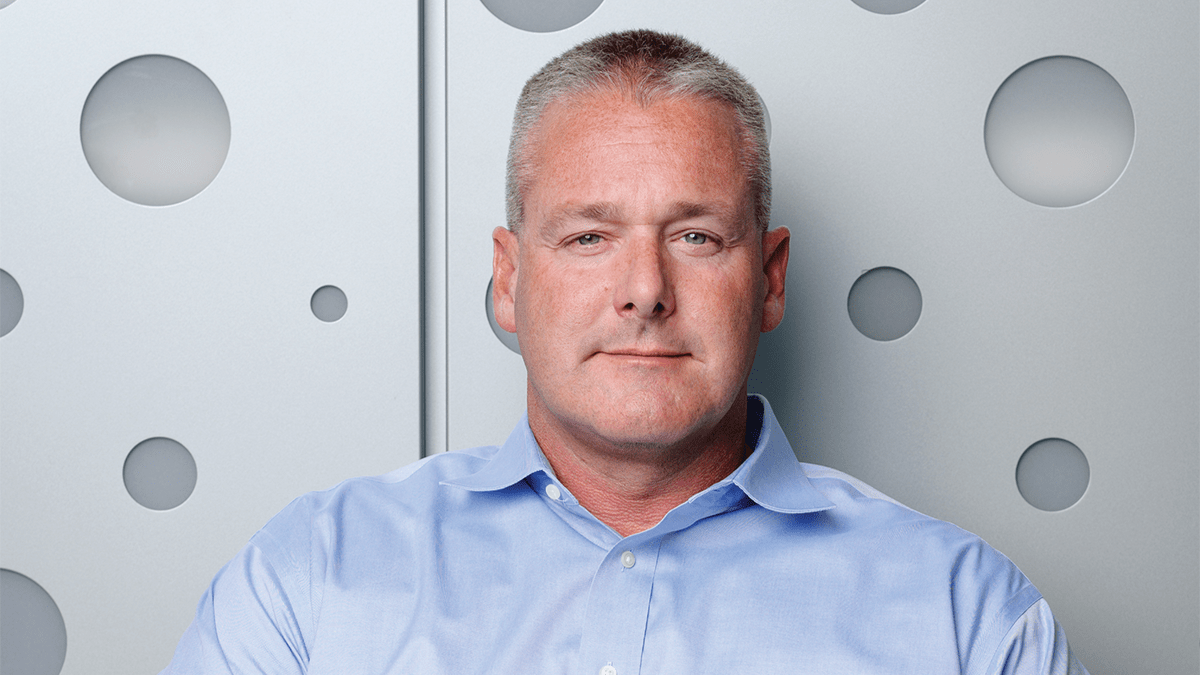
How Radiology is Becoming a Leader in Adopting AI
Few clinical areas have adopted AI tools faster than radiology, easing workloads and helping overcome a shortage of clinicians.
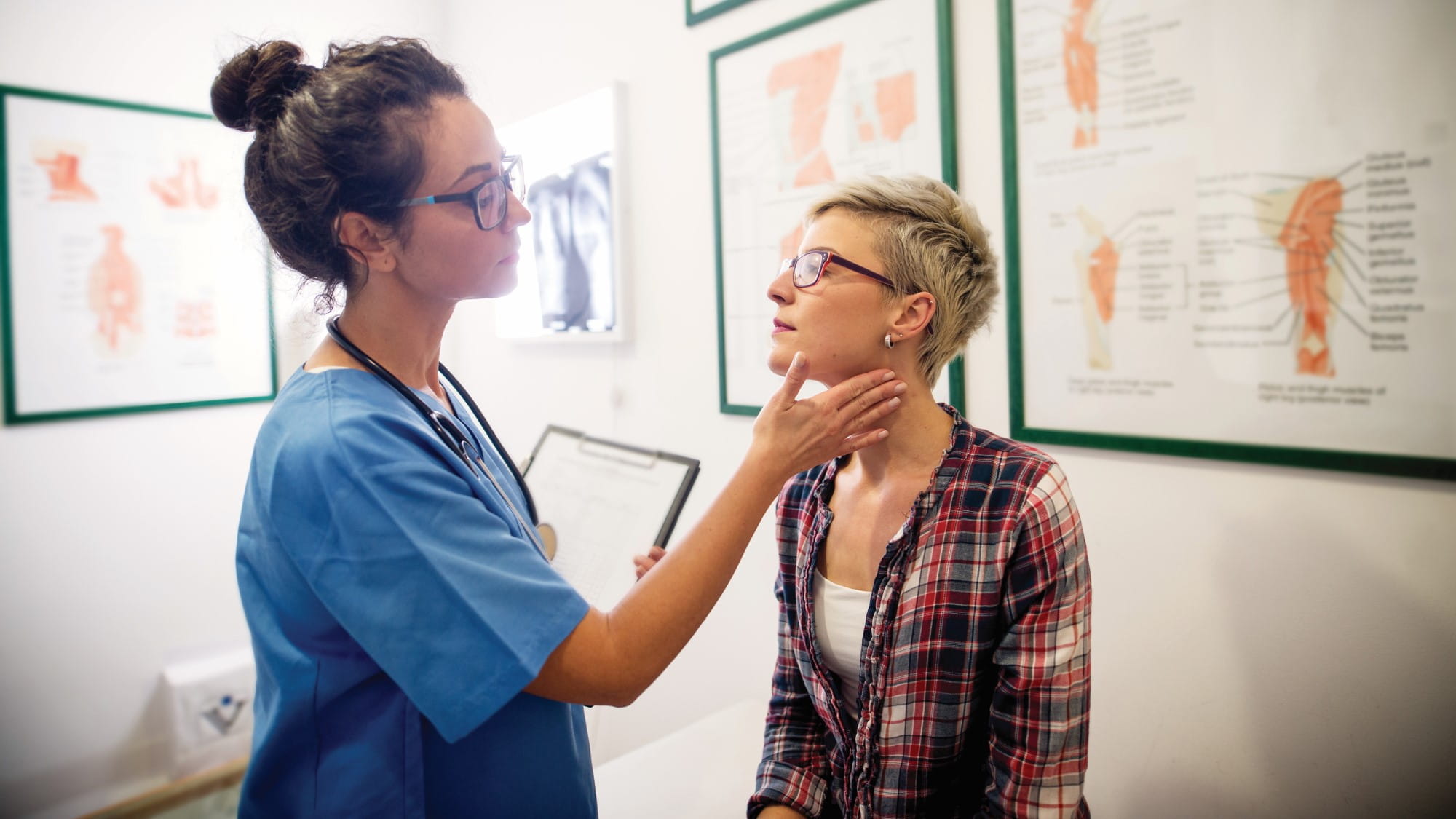
AI is Coming to an Exam Room Near You
Ambient listening interprets conversations in real-time to update electronic health records — and help physicians reconnect with their patients.
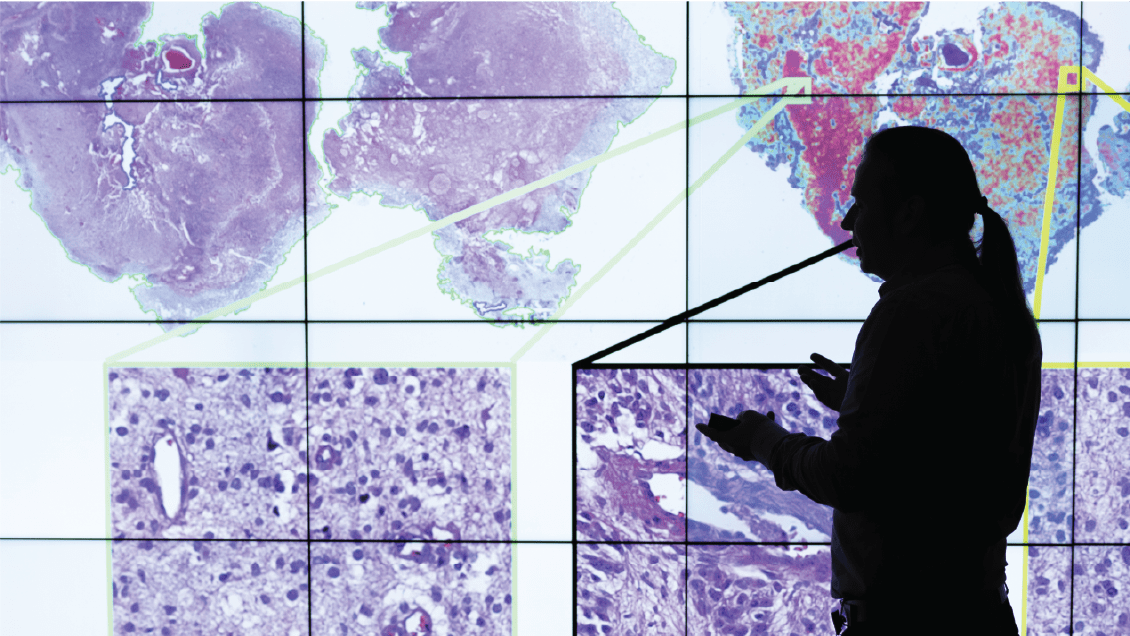
AI That Learns Without Borders
Decentralized approaches to AI make it easier for scientists to share data, protect sensitive data and develop tools that reflect the diversity of patients.
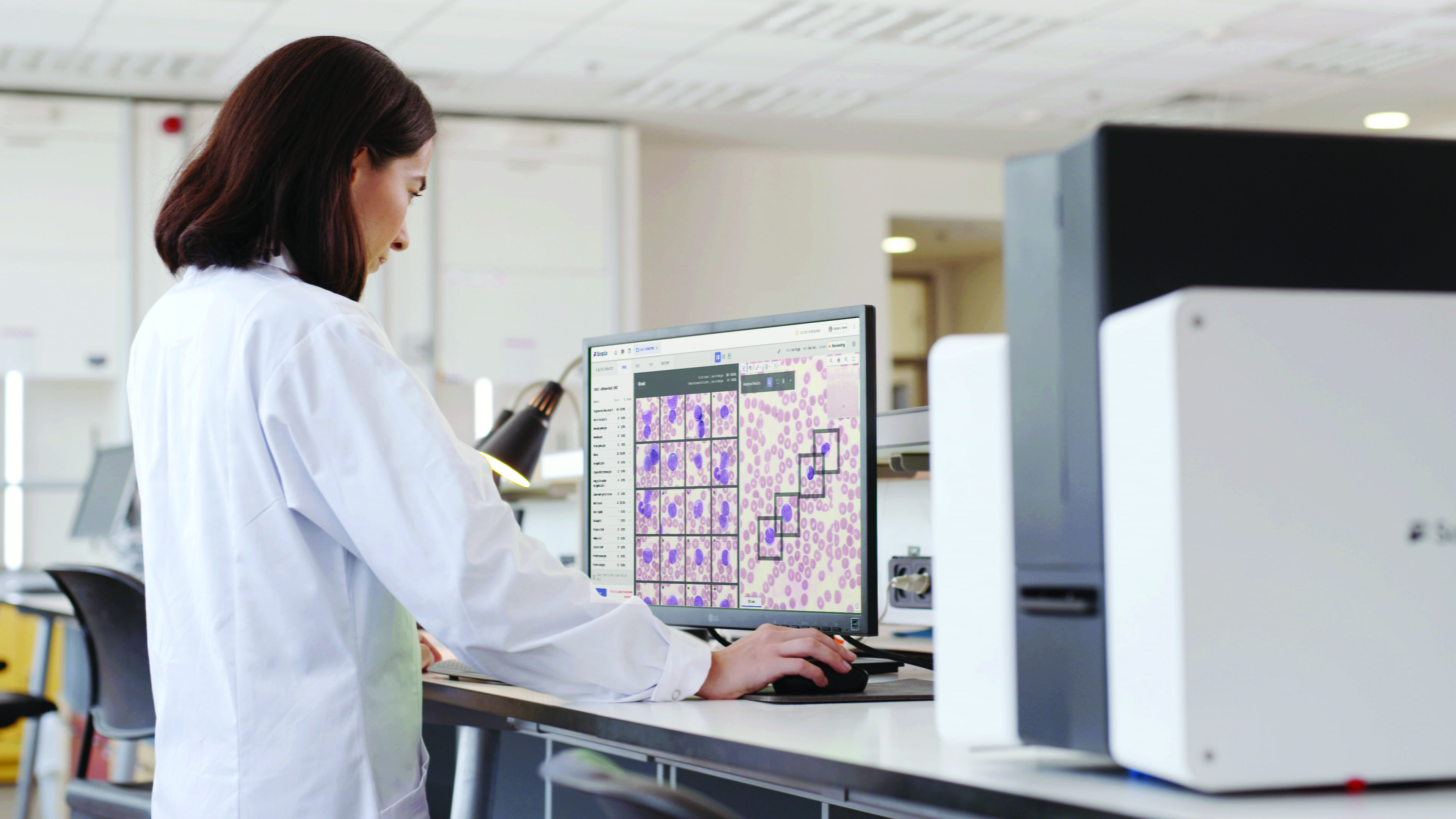
"We're on the Precipice"
AI powers tools that help pathologists spend less time counting cells and use their refined skills to make complicated diagnoses.
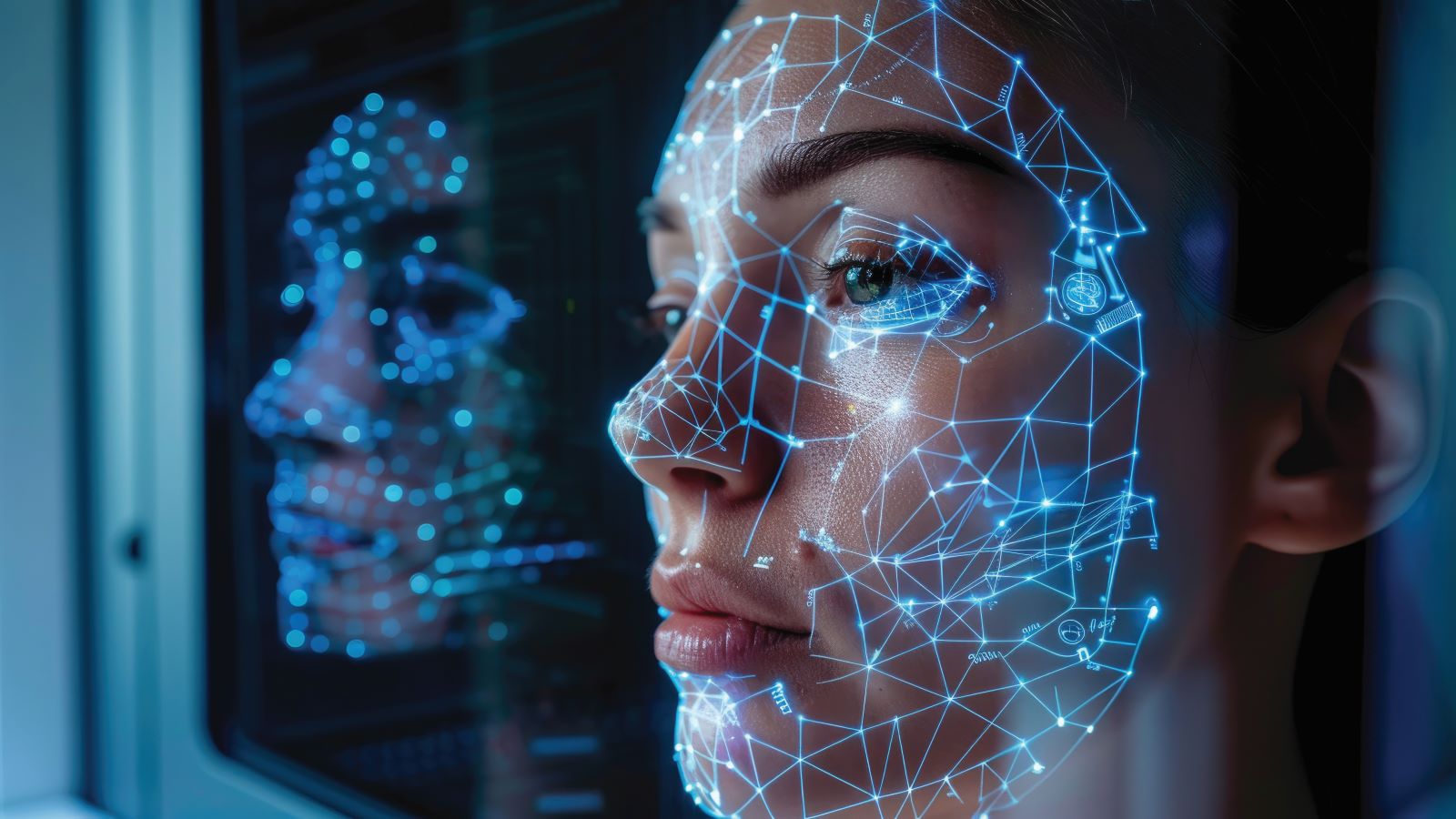
Skin in the Game
An IU researcher’s AI tool shows promise in predicting whether melanoma will return.
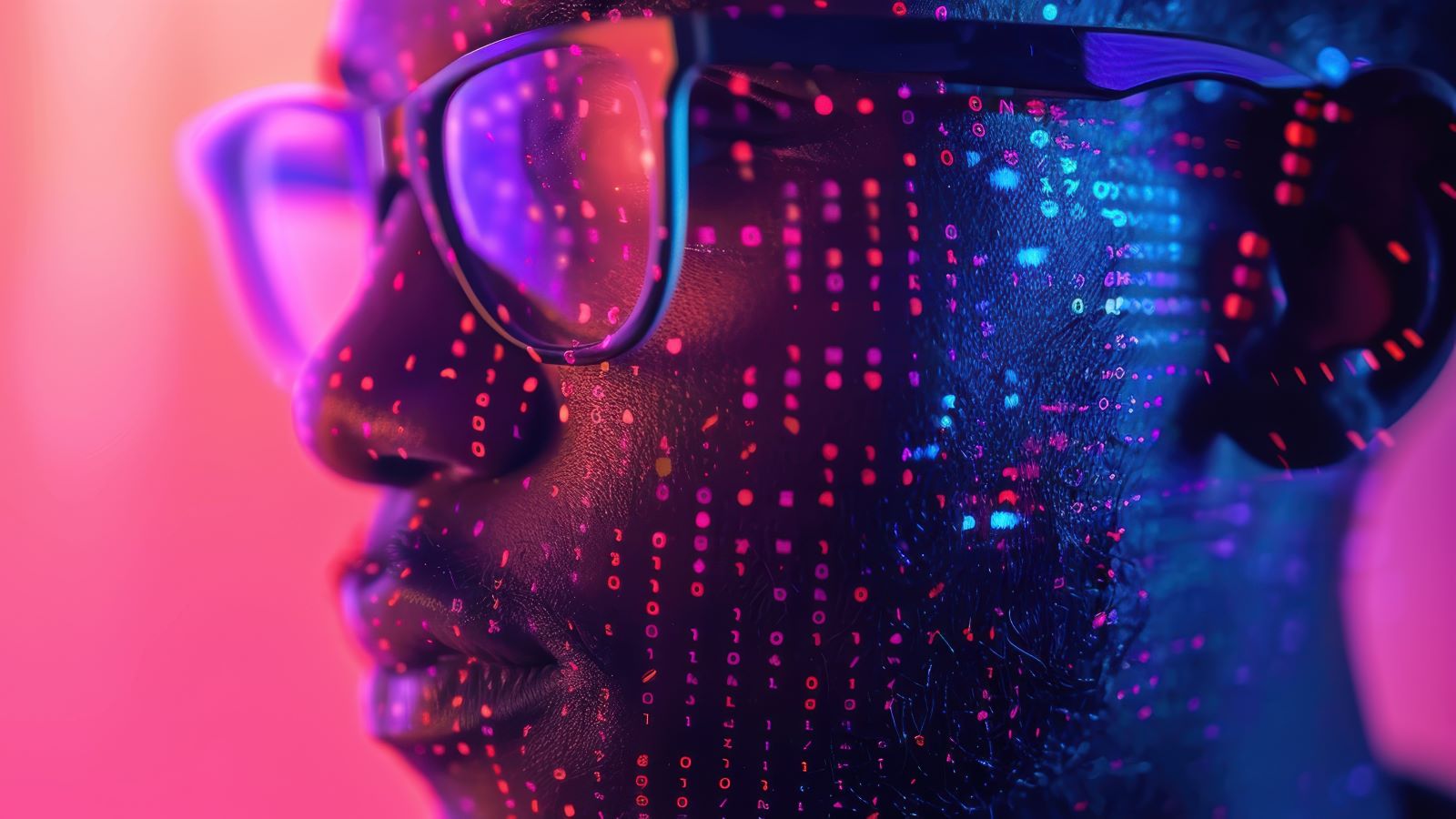
Reducing Bias in AI
In a technology hungry for data, how do we ensure it consumes good information and builds models that benefit every patient?